The C. R. and Bhargavi Rao Prize honors outstanding and influential innovations in the theory and practice of mathematical statistics, international leadership in directing statistical research, and pioneering contributions by a recognized leader in the field.
C. R. Rao
C. R. Rao (1920–2023) was one of the most influential statisticians of the 20th and 21st centuries. He held the Eberly Chair in Statistics at Penn State University from 1988 to 2001 and later served as Holder Emeritus of the Eberly Chair. He was the founding Director of the Center for Multivariate Analysis. A laureate of the U.S. National Medal of Science, Dr. Rao was recognized internationally for his pioneering contributions to statistical theory and applications. In 2023, he was awarded the International Prize in Statistics—often described as the “Nobel Prize of Statistics”—for fundamental contributions that revolutionized statistical thinking, including the Cramér-Rao inequality and Rao-Blackwell theorem. Dr. Rao’s work continues to shape disciplines as diverse as statistics, econometrics, electrical engineering, and genetics, and forms a core part of undergraduate and graduate education worldwide. His legacy as a mathematician, researcher, and teacher endures through his groundbreaking research and the generations of scholars he inspired.
The Rao Prize is awarded in odd-numbered years by the Department of Statistics at Penn State University to a nominee selected by the members of the Rao Prize Committee. Nominations should include a letter describing the nominee’s outstanding contributions to leadership and research in statistics, a current curriculum vitae, and two supporting letters. The award recipient will receive a medal, a cash prize, and an invitation to visit Penn State to give a talk.
The C. G. Khatri Memorial Lectureship and P. R. Krishnaiah Memorial Lectureship, which began as two Visiting Scholars programs in 1992, honor the memory of C. G. Khatri and P. R. Krishnaiah by inviting outstanding researchers in statistics to deliver lectures at Penn State.
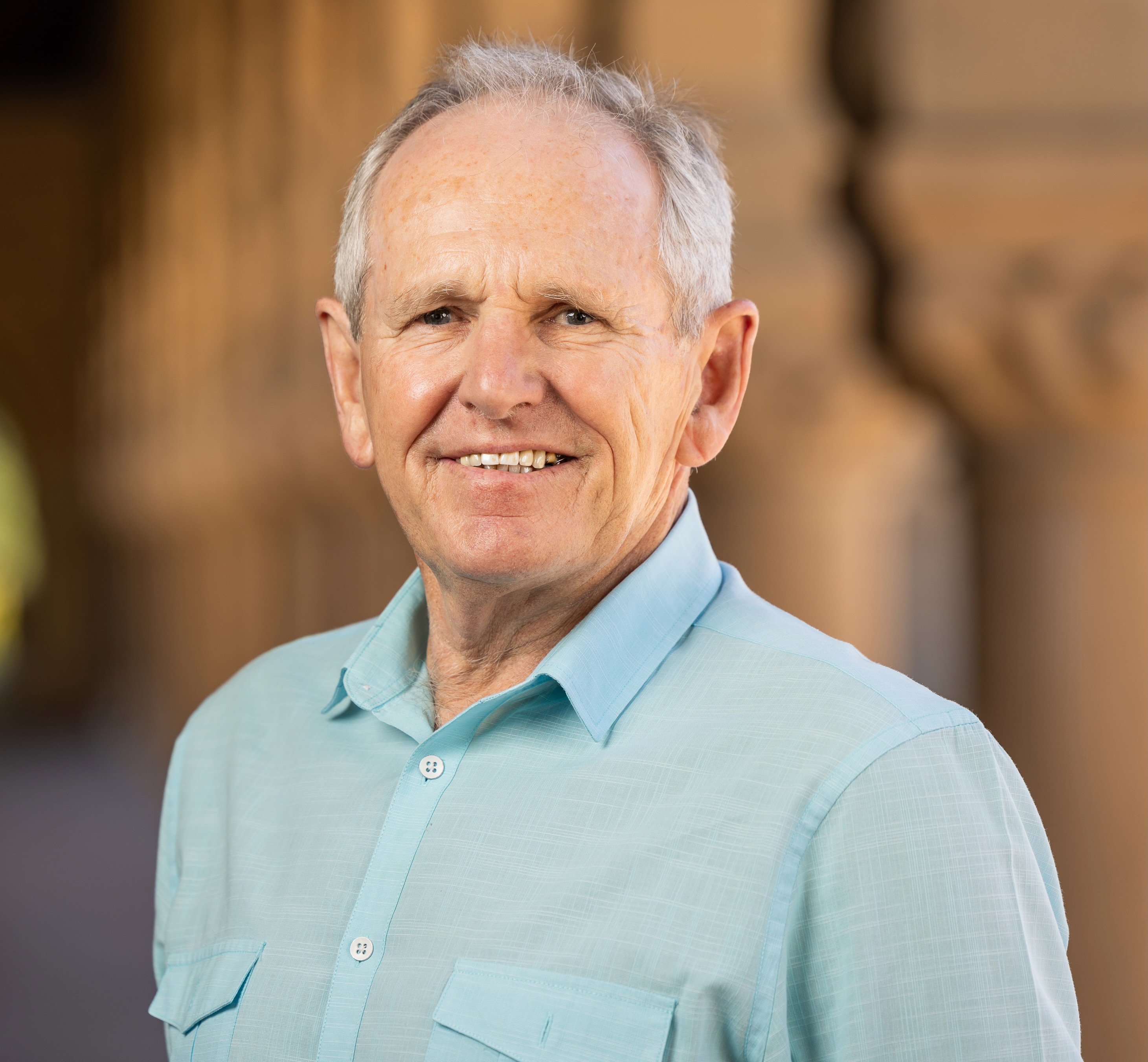
Rao Prize Winner
Trevor Hastie
Stanford University
Trevor Hastie is the John A. Overdeck Professor of Statistics at Stanford University. Hastie is known for his research in applied statistics, particularly in the fields of statistical modeling, bioinformatics, and machine learning. He has published six books and over 200 research articles in these areas. Prior to joining Stanford University in 1994, Hastie worked at AT&T Bell Laboratories for nine years, where he contributed to the development of the statistical modeling environment popular in the R computing system. He received a B.Sc. (Hons) in statistics from Rhodes University in 1976, a M.Sc. from the University of Cape Town in 1979, and a Ph.D from Stanford in 1984. In 2018 he was elected to the U.S. National Academy of Sciences. His main research contributions have been in applied statistics; he has published over 200 articles and written six books in this area: "Generalized Additive Models" (with R. Tibshirani, Chapman, and Hall, 1991), "Elements of Statistical Learning" (with R. Tibshirani and J. Friedman, Springer 2001; second edition 2009), "An Introduction to Statistical Learning, with Applications in R" (with G. James, D. Witten and R. Tibshirani, Springer 2013, second edition 2021) and "Statistical Learning with Sparsity" (with R. Tibshirani and M. Wainwright, Chapman and Hall, 2015) and "Computer Age Statistical Inference" (with Bradley Efron, Cambridge 2016, "student" edition 2021). He has also made contributions in statistical computing, co-editing (with J. Chambers) a large software library on modeling tools in the S language ("Statistical Models in S", Wadsworth, 1992), which form the foundation for much of the statistical modeling in R. His current research focuses on applied statistical modeling and prediction problems in biology and genomics, medicine and industry.
Title: Univariate-Guided Sparse Regression
Abstract
In this talk, we introduce "UniLasso'' -- a novel statistical method for sparse regression. This two-stage approach preserves the signs of the univariate coefficients and leverages their magnitude. Both of these properties are attractive for stability and interpretation of the model. Through comprehensive simulations and applications to real-world datasets, we demonstrate that UniLasso outperforms Lasso in various settings, particularly in terms of sparsity and model interpretability. Extensions to generalized linear models (GLMs) and Cox regression are also discussed. This is joint work with Sourav Chatterjee and Rob Tibshirani.
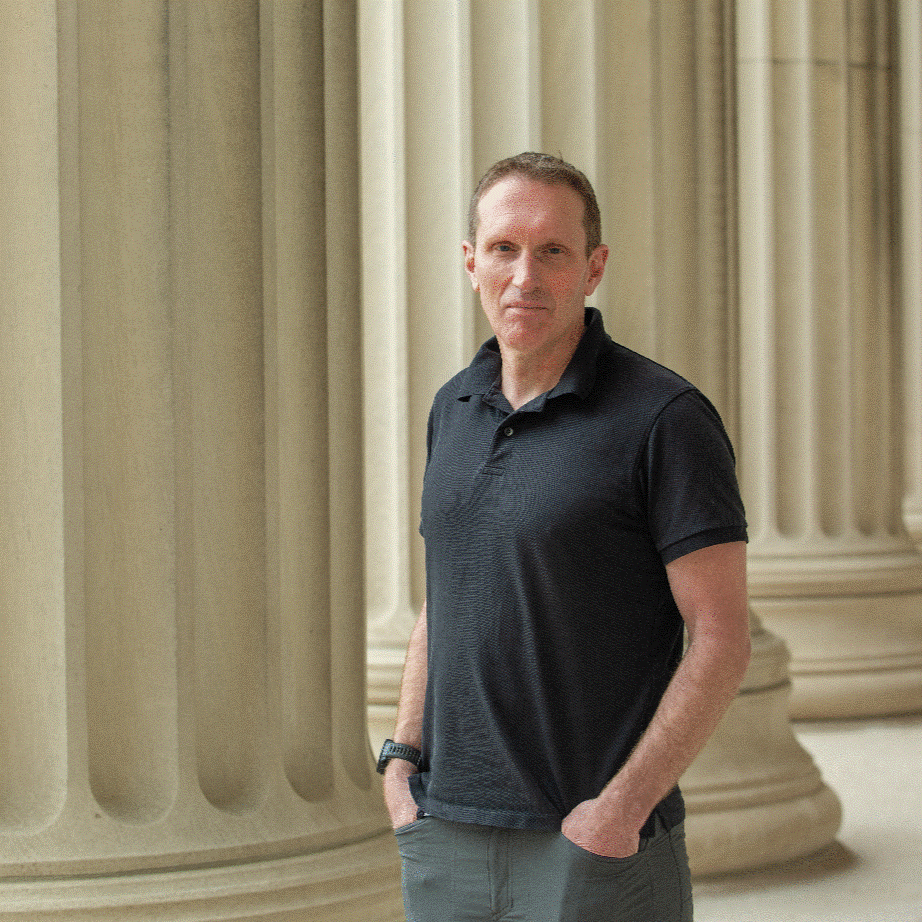
Krishnaiah Lecturer
Martin Wainwright
Massachusetts Institute of Technology
Title: New Frontiers in Statistical Prediction: Surrogate Training and Risk Prediction
Abstract
Given the explosion of data and computing resources, it is now routine to fit predictive models---among them neural nets, large-scale kernel machines, and boosted models---for complex and high-dimensional tasks. At the same time, the performance of such methods can be mysterious and have undesirable properties, such as sensitivity to distribution shift, severe mis-calibration, and other forms of non-robustness. This talk discusses some recent progress at this frontier, including a surrogate training method that can address bias and mis-calibration, and a computationally efficient method for bounding prediction error with non-asymptotic guarantees.
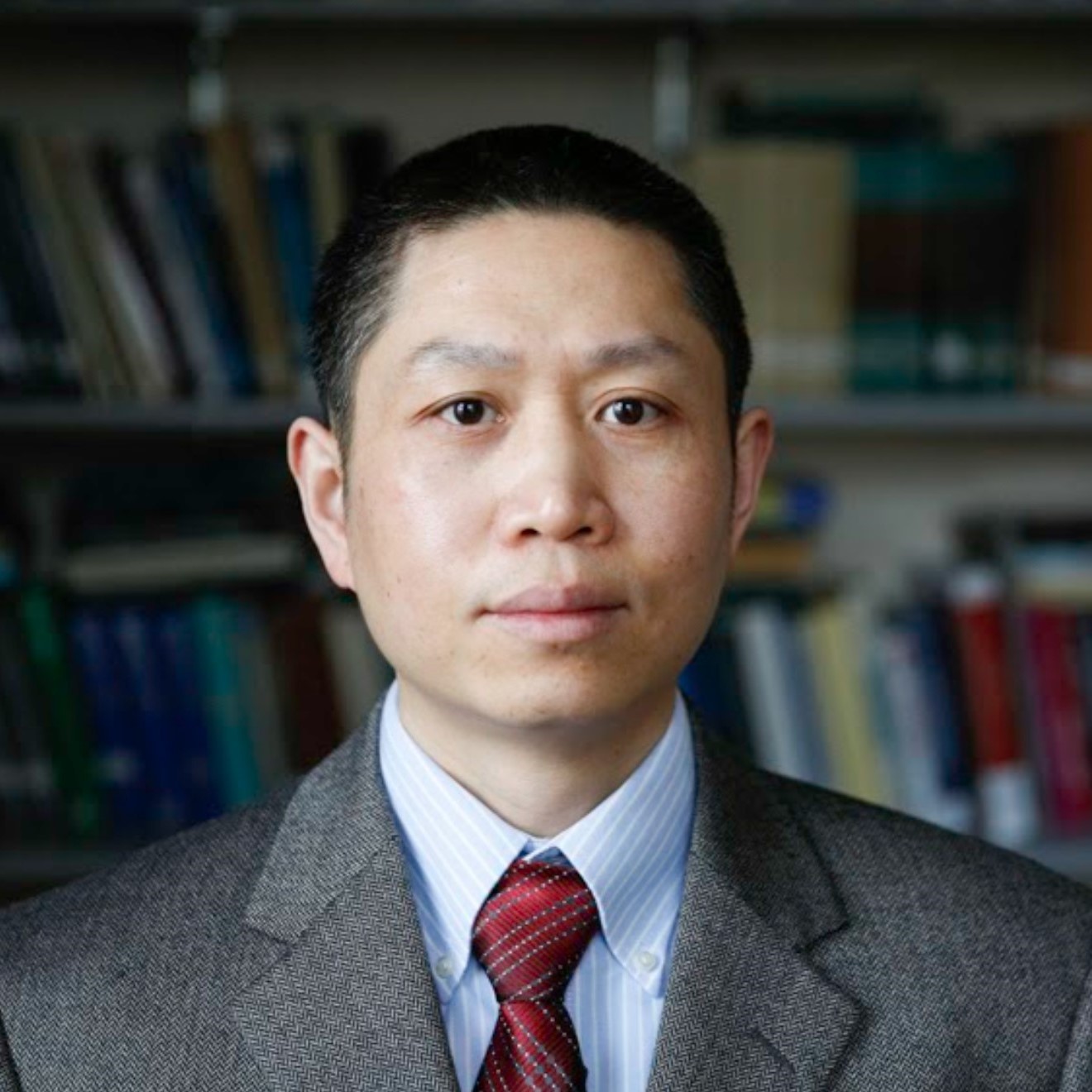
Khatri Lecturer
Hui Zou
University of Minnesota
Title: High-Dimensional Clustering via a Latent Transformation Mixture Model
Abstract
Cluster analysis is a fundamental task in machine learning. From the probabilistic modeling viewpoint, a finite mixture model is naturally suited for the distribution of data with multiple clusters, and hence model-based clustering (MBC) offers an effective solution. MBC has been successful in numerous applications. However, MBC also often underperforms when the model has significant modeling bias. We aim to design a more robust off-the-shelf MBC for high-dimensional data by reducing the model bias. To this end, we propose a novel CESME model by incorporating nonparametric latent transformations into the finite Gaussian mixture model (GMM). The inclusion of latent transformations significantly enhances the flexibility of the finite GMM without compromising interpretability. We derive a model fitting procedure for implementing the optimal clustering under the CESME model and analyze the clustering accuracy of the resulting algorithm. It is shown that the additional cost due to estimating nonparametric transformations is negligible compared with an ideal clustering algorithm with known transformations. On six benchmark single-cell RNA sequence datasets, CESME exhibits dominating advantages over existing methods in the literature.
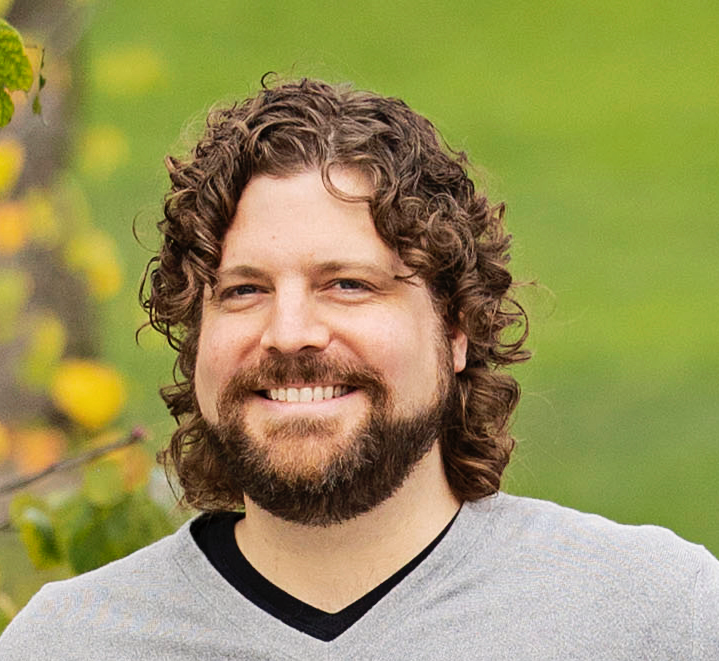
Speaker
Will Fithian
University of California, Berkeley
Title: Estimating the FDR of Variable Selection
Abstract
We introduce a generic estimator for the false discovery rate of any model selection procedure, in common statistical modeling settings including the Gaussian linear model, Gaussian graphical model, and model-X setting. We prove that our method has a conservative (non-negative) bias in finite samples under standard statistical assumptions, and provide a bootstrap method for assessing its standard error. For methods like the Lasso, forward-stepwise regression, and the graphical Lasso, our estimator serves as a valuable companion to cross-validation, illuminating the tradeoff between prediction error and variable selection accuracy as a function of the model complexity parameter.
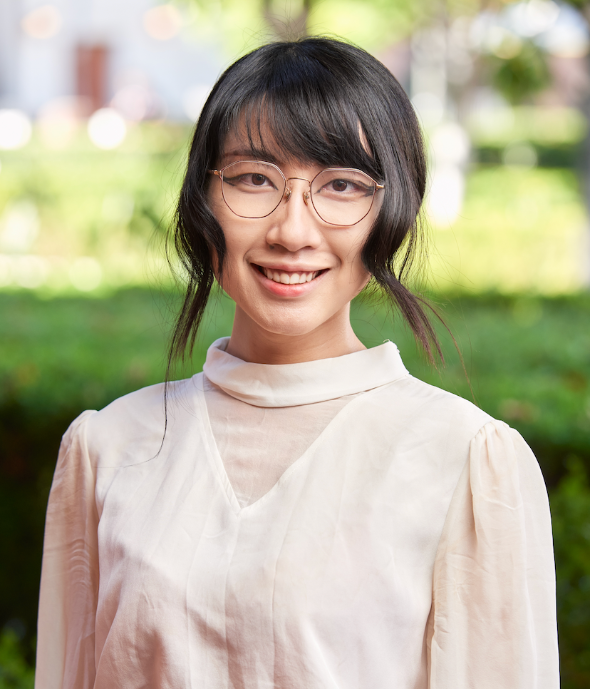
Speaker
Zijun Gao
USC Marshall Business School
Title: Trustworthy Assessment of Heterogeneous Treatment Effect Estimator via Analysis of Relative Error
Abstract
Accurate heterogeneous treatment effect (HTE) estimation is essential for personalized recommendations, making it important to evaluate and compare HTE estimators. Traditional assessment methods are inapplicable due to missing counterfactuals. Current HTE evaluation methods rely on additional estimation or matching on test data, often ignoring the uncertainty introduced and potentially leading to incorrect conclusions. We propose incorporating uncertainty quantification into HTE estimator comparisons. In addition, we suggest shifting the focus to the estimation and inference of the relative error between methods rather than their absolute errors. Methodology-wise, we develop a relative error estimator based on the efficient influence function and establish its asymptotic distribution for inference. Compared to absolute error-based methods, the relative error estimator (1) is less sensitive to the error of nuisance function estimators, satisfying a "global double robustness" property, and (2) its confidence intervals are often narrower, making it more powerful for determining the more accurate HTE estimator. Through extensive empirical study of the ACIC challenge benchmark datasets, we show that the relative error-based method more effectively identifies the better HTE estimator with statistical confidence, even with a moderately large test dataset or inaccurate nuisance estimators.
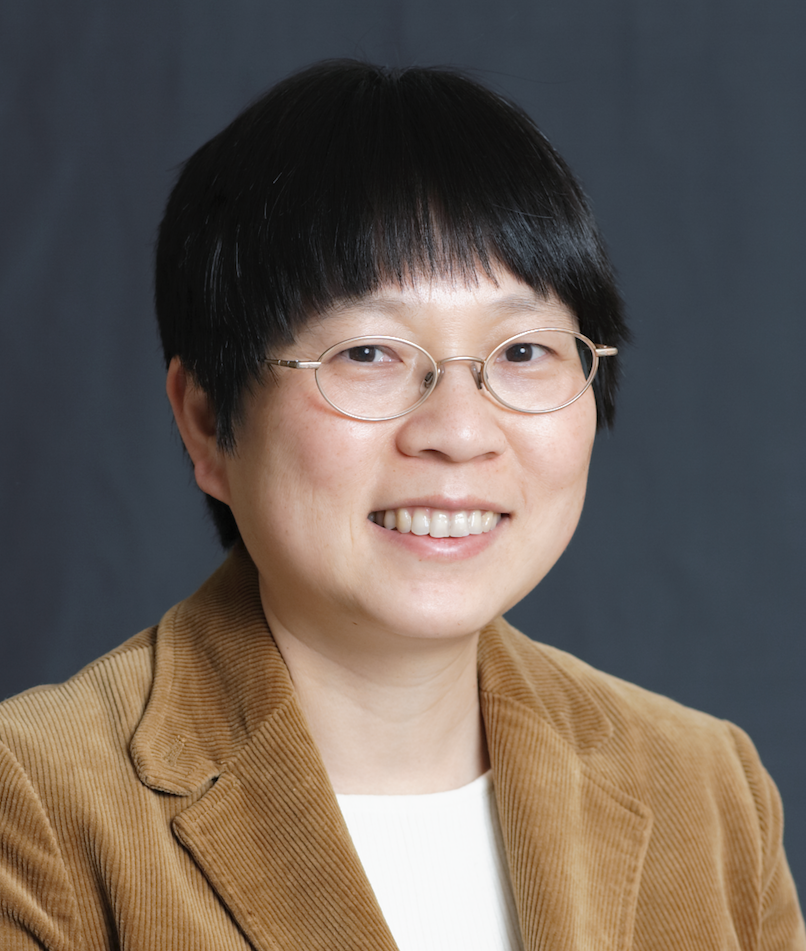
Speaker
Jia Li
The Pennsylvania State University
Title: Canonical Variates in the Wasserstein Metric Space
Abstract
We consider the classification of instances characterized by distributions over a vector space rather than single points. The Wasserstein metric provides a natural measure of distance between these distributions, which we leverage in distance-based classification methods such as k-nearest neighbors and pseudo-mixture modeling. Central to our investigation is dimension reduction in the Wasserstein metric space to improve classification accuracy and facilitate visualization. We propose a novel approach based on maximizing Fisher’s ratio—the quotient of between-class to within-class variation. The resulting discriminant coordinates, or canonical variate axes, are obtained by an alternating optimization scheme that combines optimal transport and maximization in the vector space. To handle high-dimensional data, we employ Gaussian mixture models for density estimation, avoiding reliance on discrete sample-based distributions. Additionally, we replace the Wasserstein distance with the Minimized Aggregated Wasserstein (MAW) distance to improve computational feasibility. Experimental results demonstrate the effectiveness of our method in terms of classification accuracy, robustness, and algorithmic convergence.
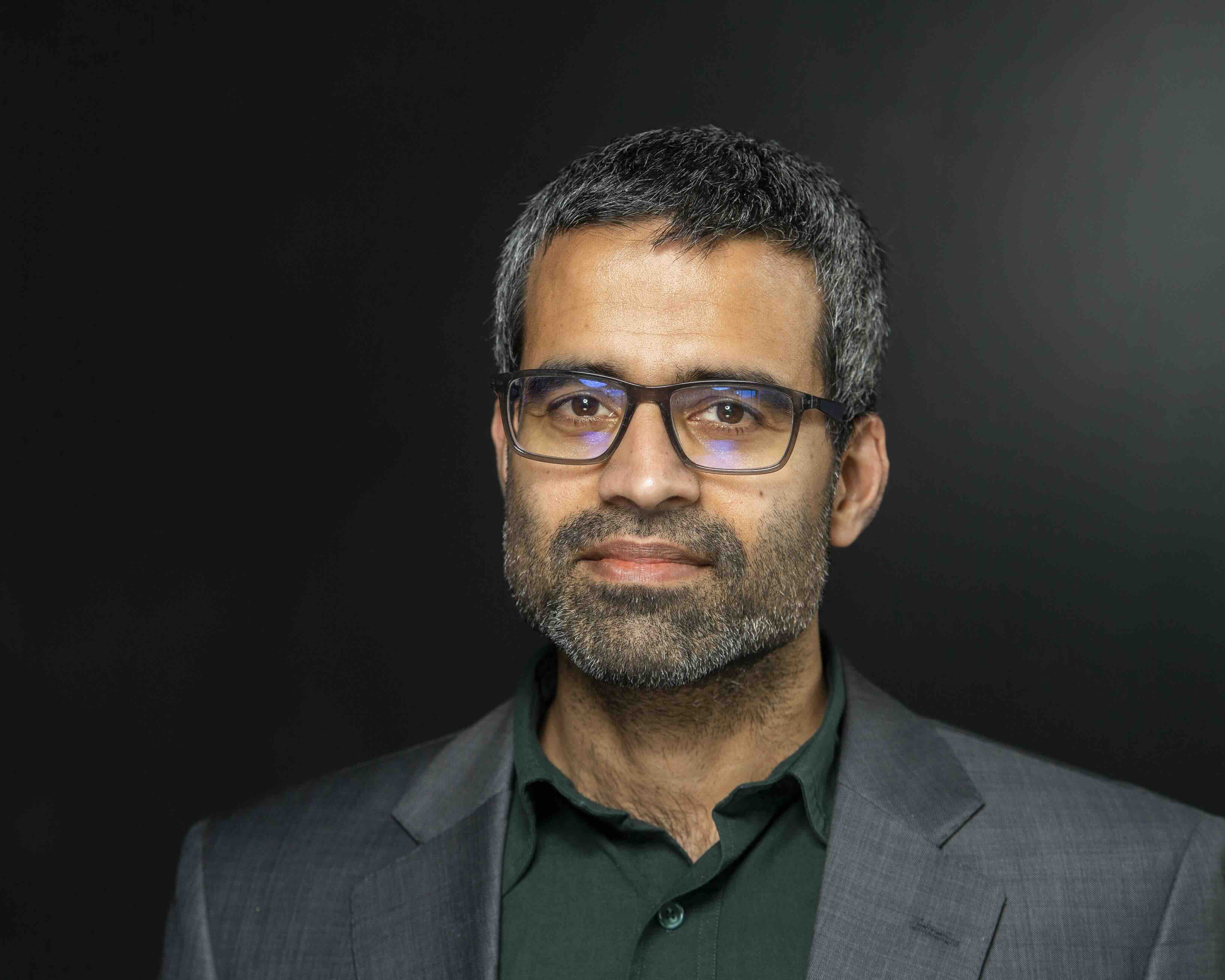
Speaker
Pradeep Ravikumar
Carnegie Mellon University
Title: Contextures: Representations from Contexts
Abstract
Despite the empirical success of foundation models, we do not have a systematic characterization of the representations that these models learn. We show that a large class of representation learning methods across a number of learning paradigms --- supervised, self-supervised, and manifold learning --- aim to extract the top-d singular functions of an operator characterized by the relationship between the inputs and a context variable. We refer to these optimal representations as contextures. One important consequence of our analytical framework is an answer to the question of whether scale is all we need for artificial general intelligence? The answer that follows from our results is no: once we can approximate the optimal contextures, scaling up model sizes further yields diminishing returns. Consequently, we posit that further improvement requires creating more useful contexts from data in a principled way, and that such contexts can lead to better representations.
Organizing Committee Contacts
8:30 a.m. - 9:00 a.m. Registration (Light Breakfast, Tea/Coffee)
9:00 a.m. - 9:10 a.m. Welcoming Remarks
9:10 a.m. - 9:20 a.m. Introduction and Award Presentation
9:20 a.m. - 10:20 a.m. 2025 Rao Prize Recipient: Trevor Hastie, Stanford University
10:20 a.m. - 10:40 a.m. Break
10:40 a.m. – 11:20 a.m. 2025 Khatri Lecturer: Hui Zou, University of Minnesota
11:20 a.m. – 12:00 p.m. Zijun Gao, USC Marshall Business School
12:00 p.m. – 2:00 p.m. Lunch and Poster Session, Huck Life Sciences Building, Verne M. Willaman Gateway to the Sciences (Third Floor Bridge)
2:00 p.m. – 2:40 p.m. Jia Li, Penn State University
2:40 p.m. – 3:20 p.m. Will Fithian, University of California, Berkeley
3:20 p.m. – 3:40 p.m. Break
3:40 p.m. – 4:20 p.m. Pradeep Ravikumar, Carnegie Mellon University
4:20 p.m. – 5:00 p.m. 2025 Krishnaiah Lecturer: Martin Wainwright, Massachusetts Institute of Technology
5:00 p.m. – 5:10 p.m. Concluding Remarks