Overview
Speaker
Aaditya Ramdas, Associate Professor, Department of Statistics and Data Science & Department of Machine Learning, Carnegie Mellon University
Moderator
Abstract
About the Speaker
Aaditya Ramdas is an Associate Professor at Carnegie Mellon University in the Departments of Statistics and Machine Learning. His work has been recognized by the Presidential Early Career Award (PECASE), the highest distinction bestowed by the US government to young scientists, a Kavli fellowship from the NAS, a Sloan fellowship in Mathematics, a CAREER award from the NSF, the inaugural COPSS Emerging Leader Award, the Bernoulli new researcher award and the IMS Peter Hall Early Career Prize, and faculty research awards from Adobe and Google. He was recently elected Fellow of the IMS, was awarded Statistician of the Year by the ASA's Pittsburgh Chapter, and will be the program chair of AISTATS 2026. See Profile
About the Moderator
Dr. Will Wei Sun is an Associate Professor of Management at Purdue University's Mitchell E. Daniels, Jr. School of Business, with a courtesy appointment in the Department of Statistics. He serves as the PhD Coordinator for Quantitative Methods and is recognized for his expertise in statistical foundations of large language models, trustworthy reinforcement learning, online decision-making in two-sided markets, and neuroimaging analysis. Dr. Sun's research has been supported by notable grants, including a \$450K award from the National Science Foundation for work on trustworthy reinforcement learning, and a \$150K grant from the Office of Naval Research focusing on statistical methods for large tensor data. Dr. Sun earned his Ph.D. in Statistics from Purdue University in 2015, following M.S. degrees in Statistics and Computer Science from Purdue and in Statistics from the University of Illinois at Chicago. He began his academic career at the University of Miami before returning to Purdue. His teaching excellence has been acknowledged with multiple awards, such as the Salgo-Noren Outstanding Master's Teaching Award (First runner-up) in 2023 and 2024, and the Distinguished Teacher award from the Krannert School of Management in 2019, 2020, 2021, and 2023. Dr. Sun is also a member of the editorial board for the Journal of Data and Dynamic Systems. See Profile
About AI, StAtIstics and Data Science in Practice
The NISS AI, Statistics and Data Science in Practice is a monthly event series will bring together leading experts from industry and academia to discuss the latest advances and practical applications in AI, data science, and statistics. Each session will feature a keynote presentation on cutting-edge topics, where attendees can engage with speakers on the challenges and opportunities in applying these technologies in real-world scenarios. This series is intended for professionals, researchers, and students interested in the intersection of AI, data science, and statistics, offering insights into how these fields are shaping various industries. The series is designed to provide participants with exposure to and understanding of how modern data analytic methods are being applied in real-world scenarios across various industries, offering both theoretical insights, practical examples, and discussion of issues.
Event Type
- NISS Hosted
Website
Location
Policy
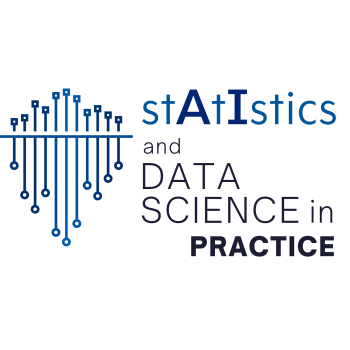