A Representational Model of Grid Cells Based on Matrix Lie Algebras
Ying Nian Wu (Department of Statistics, University of California, Los Angeles)
Abstract: A key perspective of deep learning is representation learning, where concepts are embedded in latent space and are represented by latent vectors whose units can be interpreted as neurons. In this talk, I will explain a representational model in the mammalian brain for navigation that involves grid cells and place cells. The grid cells in the mammalian medial entorhinal cortex exhibit striking hexagon firing patterns when the agent (e.g., a rat or a human) navigates in the open field. It is hypothesized that the grid cells are involved in path integral so that the agent is aware of its self-position by accumulating its self-motion. Assuming the grid cells form a vector representation of self-position, we elucidate a minimally simple recurrent model for path integral, which models the change of the vector representation given the self-motion, and we uncover two matrix Lie algebras and their Lie groups that are naturally coupled together. This enables us to connect the path integral model to the dimension reduction model for place cells via group representation theory of harmonic analysis. By reconstructing the kernel functions for place cells, our model learns hexagon grid patterns that characterize the grid cells. The learned model is capable of near perfect path integral, and it is also capable of error correction. Joint work with Ruiqi Gao, Jianwen Xie, and Song-Chun Zhu.
About the Speaker
Dr. Ying Nian Wu is currently a professor in the Department of Statistics, UCLA. He received his A.M. degree and Ph.D. degree in statistics from Harvard University in 1994 and 1996 respectively. He was an assistant professor in Department of Statistics, University of Michigan from 1997 to 1999. He joined UCLA in 1999. He has been a full professor since 2006. Wu’s research areas include Generative Modeling, Representation Learning, Unsupervised Learning, Computer Vision, Computational Neuroscience, and Bioinformatic
Final Series Speaker is:
September 11, 2020, 10:30 - 11:30 am ET
Ruslan Salakhutdinov (Machine Learning Department, School of Computer Science, Carnegie Mellon University)
"Integrating Domain-Knowledge into Deep Learning"
We are able to take advantage of the online platform this year to broadcast these talks live via both Zoom meetings.
Detailed information about connecting and other schedule information are available at the webinar series website.
Event Type
- NISS Sponsored
Host
Sponsor
Website
Location
Policy
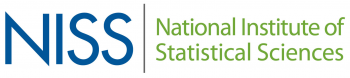