Integrating Domain-Knowledge into Deep Learning
Ruslan Salakhutdinov, (Machine Learning Department, School of Computer Science, Carnegie Mellon University
Abstract
I will first discuss deep learning models that can find semantically meaningful representations of words, learn to read documents and answer questions about their content. I will introduce methods that can augment neural representation of text with structured data from Knowledge Bases (KBs) for question answering, and show how we can answer complex multi-hop questions using a text corpus as a virtual KB. In the second part of the talk, I will show how we can design modular hierarchical reinforcement learning agents for visual navigation that can perform tasks specified by natural language instructions, perform efficient exploration and long-term planning, learn to build the map of the environment, while generalizing across domains and tasks.
About the Speaker
Dr. Ruslan Salakhutdinov is a UPMC professor of Computer Science in the Machine Learning Department, School of Computer Science at Carnegie Mellon University. He received his M.S. degree and Ph.D. degree both in Computer Science from the University of Toronto in 2003 and 2009 respectively. He works in the field of statistical machine learning. His research interests include Deep Learning, Probabilistic Graphical Models, and Large-scale Optimization. He has received numerous awards including Google Focused Award, Microsoft Research Faculty Fellowship, and Sloan Research Fellowship.
We are able to take advantage of the online platform this year to broadcast these talks live via both Zoom meetings.
Detailed information about connecting and other schedule information are available at the webinar series website.
Event Type
- NISS Sponsored
Host
Sponsor
Website
Location
Policy
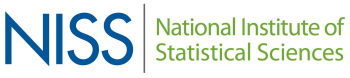