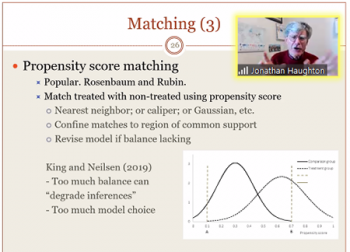
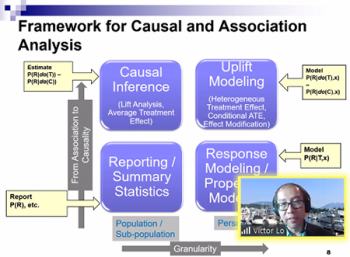
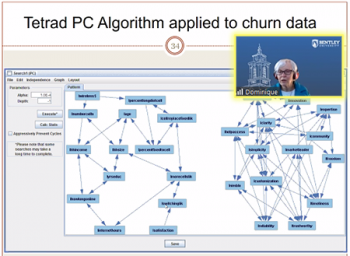
Three distinguished statisticians /data scientists teamed up to provide three presentations related to topics that are of great interest these days in the business world: causality and causal inference, uplift modeling and directed acyclic graphs. The presenters for NISS’s fourth Essential Data Science for Business tutorial were Jonathan Haughton (Suffolk University), Victor Lo (Fidelity Investments) and Dominique Haughton (Bentley University). Each presenter in turn worked to describe, in detail, the business applications of each of these approaches.
The Topics
Jonathan Haughton was the first to provide his remarks. Essentially his goal was to dive into the details of what it means to evaluate the impact of an intervention. Jonathan did this by walking through a number of examples related to the different approaches that have been used in business to go about solving this type of problem. These include different types of random assignment of treatments, a couple of approaches involving regression, and various matching techniques.
Victor Lo concentrated his comments on a relatively new method labeled ‘Uplift Modeling’, which might also now be recognized by other labels such as subgroup analysis in statistics, conditional average treatment effect (CATE) in economics, and others in other fields of study. After describing various contexts that established the need for this type of approach, Victor reviewed a couple of different ways of going about uplift modeling including the two-model approach, the treatment dummy approach and the four-quadrant method. From here he dove into how one might go about using uplift modeling and causal inference in non-randomized experiments, providing a number of examples of this in business contexts.
The third presenter in this tutorial, Dominique Haughton, focused her attention on the use directed acyclic graphs (DAGs) in causal business analytic contexts. While randomized experiments are not always possible, Dominique offered DAGs as a way to help. Essentially, in a causal situation an important question to investigate is the direction of the causality. In using DAGs Dominique established that this approach can infer causality but under certain restrictive assumptions. From here she walked through a number of different examples to demonstrate the value that DAGs can bring to the table. At the same time, she showcased a number of different software packages that were designed for this purpose include Tetrad IV, GeNIe and BayesiaLab.
Access to Materials
Wow – so many details! Models, frameworks, methods, software, examples! If you were not able to attend this live session you can still access a recording of the session along with links to the slides that the three presenters used during this session. Use the Registration Option "Post Session Access" on the event webpage, pay the $35 fee, and NISS will provide you with access to the materials for this session. Or register for the full series of ten tutorials, and NISS will provide all the links as well.
What’s Up Next?
Mark your calendars for December 2, 2020 from 1 to 4 pm. Ming Li (Amazon) will present on "Deep Learning". This is the final fall tutorial of the Essential Data Science series for the Fall.
Register for this event today!
Here are the topics that will presented beginning in January, 2021.
- "Prescriptive Analytics and Optimization"
- "Unstructured Data Analysis"
- "Social Sciences and Data Science Ethics"
- "Domain Knowledge and Case Studies"
- "Analytical Consulting, Communication and Soft Skills"
NISS will provide details for registering and attending these sessions on the NISS website. Coming soon!