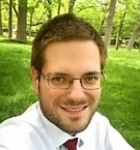
When Luca Sartore (NISS Research Fellow) joined NISS and the Research and Development Division of the National Agricultural Statistics Services (NASS) in 2015, his first research project addressed the problem of ensuring consistency for totals across different levels of aggregation (at county, state and/or national levels) since integer weights are required. A problem arose because separately rounding of the each unit’s weights led to totals for small units (e.g., county or state) that did not equal the totals at a higher level (e.g., state or nation). This work, with Cliff Spiegelman as his mentor at NASS, has now gone into production and has been adapted for additional NASS surveys.
A Calibration Method for Benchmarking with Integer Weights
The National Agricultural Statistics Service (NASS) relies on US Census of Agriculture data, and employs calibration methods to address tabulation-consistency issues. NASS has historically used rounded calibrated weights to address these issues across different levels of aggregation rather than rounding the tabulated totals.
Until 2017, NASS’s rounding method of choice was based on stochastic algorithms then considered to be state-of-the-art in the survey statistics literature. These algorithms (e.g., the cube method) produced integer calibration weights for agricultural data without impacting the estimated number of farms. However, the rounding process did not account for relationships implicit in the calibration constraints; consequently, most (if not all) administrative benchmarks were not preserved.
Improving the rounding process and providing optimal integer calibrated weights on all responding records required a reconsideration of calibration benchmarks as a multi-objective optimization problem.
As a starting point for an iterative algorithm, a priority ordering was defined for processing the initial, non-integer weights based on relative contribution to estimated totals. The gradient of an objective function then was used to force the estimated totals to be as close as possible to the administrative benchmarks. This
first version of the iterative algorithm
(“Integer Calibration”) yielded rounded weights that were better than those from the old stochastic rounding method. This new approach was computationally appealing, but not a full solution.
The first adjustment to the algorithm replaced the standard raking method and used an integer lattice for computation, which did improve the quality of the estimates.
The second revision used a continually updated priority index, based on the gradient of the objective function. This minimized the number of operations required for simultaneous minimization of all relative errors (differences between estimated totals and calibrated benchmarks).
With these revisions, the new Integer Calibration method was again evaluated relative to the old raking methods used for the 2012 US Census of Agriculture. Actual 2012 Census data was used to compare performance for the Integer Calibration method against 2012 calibration benchmarks. The new method attained more calibration benchmarks than the earlier method; and correlations were higher between the initial dual-system-estimation weights and the final ones for the new method.
Additional computational improvements further reduced processing time for large datasets. First, well-defined benchmarking equations led to faster evaluation of the calibration errors. Second, sparse matrix representations considerably reduced the amount of memory required to store the data. Third, L1-normed objective functions resulted in faster evaluations of gradient by using recursive updating formulas. With these improvements, optimal integer weights are now computed within minutes rather than hours.
The Integer Calibration method was fully implemented for the 2017 US Census of Agriculture, allowing NASS analysts to quickly evaluate the quality of the estimates across several levels of aggregation. Based on this success, NASS has applied similar methodology to the Local Food Survey and the Labor Survey as well.