Research Project
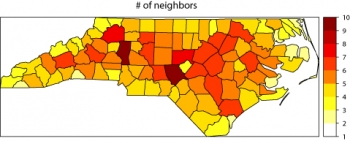
Syndromic surveillance uses syndrome (a specific collection of clinical symptoms) data to monitor for indications of a potential or incipient disease outbreak. Other advanced surveillance systems have been implemented globally for early detection of infectious disease outbreaks and bioterrorist attacks. However, such systems are often confronted with the challenges to (1) incorporate situation specific characteristics such as covariate information for certain diseases; (2) embed the spatial and temporal dynamics of the disease; and (3) provide analysis and visualization tools to help detect unexpected patterns. New methods to improve the overall detection capabilities of these systems must also minimize the number of false alerts (false positives) that have extensive ramifications and broad social impact.
The model developed is epidemiological and draws on a Gaussian Markov random field (GMRF) formulation with spatio-temporal conditional autoregressive (CAR) modeling. Comparative advantages of this model include timely detection of outbreaks, robust inference to model misspecification, reasonable prediction performance, as well as attractive analytical and visualization tools to assist public health authorities in risk assessment. Extensive simulation studies and synthetic data generated from a dynamic SIR model demonstrate that the model is capable of capturing outbreaks rapidly, while still limiting false positives. The prototype for implementation defined a network of North Carolina counties where the basic model incorporates the irregular spatial geographical relationships between the counties. The implementation utilized daily local flu counts to distinguish an outbreak of flu in a county from background counts.
Develop an inference model for determining the location of outbreaks of epidemics in a network of nodes and carry out a prototype implementation.
In parallel with SAMSI’s 2009-10 program, Space-Time Analysis for Environmental Mapping, Epidemiology and Climate Change, NISS assembled a multi-institutional team based at NISS who simultaneously participated in the SAMSI program: Alan Karr (NISS), Jian (Frank) Zou (NISS postdoc), David Banks (Duke), Gauri Datta (U. of Georgia), Matt Heaton (NCAR), Jim Lynch (U. of South Carolina) and Francisco Vera (ESPOL and Clemson).