[Please Note: The Essential Data Science for Business: Causal Inference and Uplift Modeling has already occurred.
Go to the News Story for this event to read about what happened., or...
Missed this Event? To gain access to the recording of this event along with links to supporting files and information, complete the Registration Option for "Post Session Access" on the right hand side of this webpage.]
The tutorials in this NISS series involve the Top 10 analytics approaches of the key topics that are used in business today! Students and faculty, these are perhaps the top ten most important and practical topics that may not be covered in your program of study. (Review the Overview Presentation about all 10 Sessions).
Causal Inference and Uplift Modeling
Overview - Updated!
In this tutorial, we will discuss causal inference and uplift modeling, from statistics, econometrics, data science, and artificial intelligence perspectives. We will first introduce statistical and econometric approaches to causal inference including the potential outcomes approach and various key econometric methods. Next, we will introduce the concept of uplift modeling and various modeling techniques that can be implemented with standard data science software. We will also integrate uplift modeling with causal inference for observational data. Last but not least, we will introduce directed acyclic graph or causal Bayesian network, an artificial intelligence based approach for identifying possible causal structure and estimating causal relationships. A variety of practical examples from the industry and education will be used to illustrate the techniques.
Instructors
Victor Lo (Fidelity Investments), Dominique Haughton (Bentley University) and Jonathan Haughton (Suffolk University)
Goals
NISS is interested in sharing knowledge. To this end, these webinars have been geared to provide practical information that you can use tomorrow. Examples, projects and code sharing are a part of these sessions wherever possible.
Series Prerequisites
Participants require a working knowledge of probability distributions, statistical inference, statistical modeling and time series analysis as a prerequisite. Students who do not have this foundation or have not reviewed this material within the past couple of years will struggle with the concepts and methods that build on this foundation.
Registration
Select a registration/payment option above the 'Register for this Event' button ($35 for this Data Science Essentials tutorial session, $250 for all 10 Essential Data Science for Business tutorial sessions. Can attend this live session? Post Session Access to tutorial materials and recording can be obtained for $35 after the event is over.). NISS Affiliates, (https://www.niss.org/affiliates-list), please send an email to officeadmin@niss.org.). Notifications: You will recieve an email that comes immediately to let you know you paid. Links to the event will come via email the day before and one hour prior to the actual session.
Agenda
About the Instructors
Victor S.Y. Lo is a seasoned Big Data, Marketing, Risk, and Finance leader with over 25 years of extensive consulting and corporate experience employing data-driven solutions in a wide variety of business areas, including Customer Relationship Management, Market Research, Advertising Strategy, Risk Management, Financial Econometrics, Insurance Analytics, Product Development, Healthcare Analytics, Operations Management, Transportation, and Human Resources. He is actively engaged with causal inference and is a pioneer of Uplift/True-lift modeling, a key subfield of data science.
Dominique Haughton (Ph.D. MIT 1983) is Professor of Mathematical Sciences and Global Studies at Bentley University in Waltham, Massachusetts, near Boston, and Affiliated Researcher at the Université Paris 1 (Pantheon-Sorbonne) and at the Université Toulouse 1. Research interests include applied statistics, business analytics, global analytics, music analytics, data mining, and model selection. Professor Haughton’s work concentrates on how to best leverage modern analytics techniques in order to address questions of business or societal interest. United States co-Editor of Case Studies in Business, Industry and Government Statistics (CSBIGS). Author of three monographs, a Springer brief, and of over seventy articles which have appeared in journals such as The American Statistician, Computational Statistics and Data Analysis, Journal of Interactive Marketing, Telecommunications Policy, Economic Development and Cultural Change, Studies in Family Planning, Journal of Population Economics, Journal of Biosocial Science, Annals of Statistics, Sankhya, Journal of Statistical Computation and Simulation, Communications in Statistics, Statistica Sinica. Ecole Normale Supérieure Graduate. Fellow of the American Statistical Association. Elected to the Council of the International Statistical Institute.
Jonathan Haughton (Ph.D. Harvard 1983) is Professor and Chair of Economics at Suffolk University, Boston. A specialist in the areas of economic development, poverty, and taxation, and a prize-winning teacher, he has lectured, taught, or conducted research in over two dozen countries on five continents. His widely-cited Handbook on Poverty and Inequality (with Shahidur Khandker) was published by the World Bank in 2009; and Living Standards Analytics (with Dominique Haughton) was published by Springer in 2011. He has published 50 articles in scholarly journals, as well as a score of book chapters and over a hundred reports. His recent work with the National Institute of Statistics of Rwanda involves the measurement and analysis of poverty there.
Event Type
- NISS Hosted
- NISS Sponsored
Host
Sponsor
Cost
Location
Policy
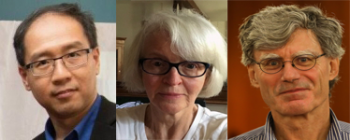